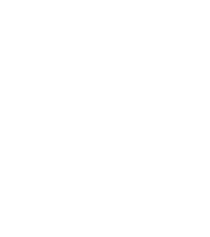
The Progress Artificial Neural Network (PANN™) is our new incomparably fast-trained artificial neural network with architecture closely emulating its biological prototype.
Speed test
In classical neural networks, training is based on various versions of the ‘gradient descent’ method, widely used in mathematics, which requires a huge number of iterative computation cycles, while the training time grows exponentially with the amount of data used for training. In addition, one of the most unpleasant properties of such networks is that it’s almost impossible to ‘update’ their training or ‘retrain’ them – any change, no matter how small, in a system that has already been trained for a long time requires an equally long new training.
Because of all this, building and running such neural networks requires the use of very powerful computers for training, a lot of highly paid programmers, a lot of expensive computer time, and electricity. Consequently, this leads to very expensive and slow developments in the advent of improving such networks, due to the long testing times of experimental iterations. That’s exactly why such developments are currently almost completely monopolized by industry giants.
The Omega neuron™ made it possible to apply a fundamentally new training method that doesn’t require many iterations, providing an almost linear dependence of PANN™ training time on the amount of data. The more difficult the task and the larger the amount of data, the greater the gap in the learning rate compared to any other modern (convolutional, etc.) neural network. This will allow for the very rapid development of such networks and products based on them, both replacing existing ones and creating completely new ones.
Most people don’t understand what AI is and, just in case, they fear it, like they do everything unknown. But artificial intelligence is just the ability of artificial systems to perform some intellectual functions. Ancient animal traps were already the simplest AI devices. Today we are surrounded by a lot of smart devices – from the thermostat that regulates the temperature in the house to airplane autopilots, programs like Word or Excel, etc. However, it’s impossible to create a full-fledged AI with software alone. Those who program these systems cannot always foresee all the situations that may arise in real life. And classical neural networks are unsuitable for this task, since they cannot learn in real time.
We can expect the emergence of many PANN™ based ‘smart servants’ that will replace ‘hard programs’ or will control them in the interests of their ‘master’. These will be systems to which one could simply ‘explain’ what needs to be done, and they would determine how best to do it, how to do it within the law, how to ensure the safety of their owner and other people, etc.
The use of PANN™ will greatly reduce the cost of creating and operating computer centers. At the same time, the reliability of data storage and protection from hackers and viruses will increase… All this is of particular importance for the implementation of large and extra-large databases, as well as indexed and non-indexed search systems in those databases. PANN™ would also be very useful in the development of unmanned systems for all types of transport.
The first artificial neural network was the universal perceptron. It didn’t work very well for specific tasks, however. So for 60 years, development efforts went mainly into the creation of specialized neural networks ‘honed’ for solving specific intellectual problems.
There are many more or less narrowly specialized neural networks – cognitrons, neocognitrons, Hopfield, Kohonen, Kosko, Jordan Elman, Boltzmann networks, recurrent networks, resonance networks, deep learning networks, convolutional networks, etc. Bayesian networks of trust and networks of cellular automata are also close to them.
PANN™ allows us to reproduce the functions of any of these networks, all while doing it much more efficiently. PANN™ also allows to create new products, for example, highly efficient personal training systems, a ‘personal doctor’, or even an ‘Alter Ego’ (second self, the ideal secretary and agent on computer networks), etc.
We are constantly expanding our intellectual property portfolio by filing new patent applications.
2013
Dmitry shared his idea with his TRIZ teachers in the US, Boris Zlotin and Vladimir Proseanic. Together they founded Progress Inc. to further develop the new technology, test it, and patent it. First tests carried out.
2014
After running more tests and filing Provisional Patent Applications, work begins to protect Progress Inc.’s Intellectual Property with patents around the world
2015
Progress Inc. prepared and filed its first US patent application in September 2015. The same year we took part in the International AI Conference in Las Vegas.
2017
Progress Inc. received the second US patent for the PANN™ technology detailing ways of implementing PANN™ on different platforms.
2018
First real-world test of the PANN™ technology through integration into a commercial product previously based on a standard neural network. Work begins on filing for patents in Japan, China, South Korea and other countries.
2019
Progress Inc. received the third US patent for the PANN™ technology detailing a new matrix training method for PANN™ networks.
2021
Progress Inc. is preparing to launch a benchmarking and experimentation platform to allow the general public to test PANN™ capabilities.

…Lorem ipsum dolor sit amet, consectetur elit sed do eiusmod tempor incididunt ut labore et dolore magna aliqua enim

…Lorem ipsum dolor sit amet, consectetur elit sed do eiusmod tempor incididunt ut labore et dolore magna aliqua enim

…Lorem ipsum dolor sit amet, consectetur elit sed do eiusmod tempor incididunt ut labore et dolore magna aliqua enim